The models after pruning can be used as is. Other methods require computationally expensive retraining or a weight update process.
Paper: https://arxiv.org/abs/2306.11695
Code: https://github.com/locuslab/wanda
Excerpts:
The argument concerning the need for retraining and weight update does not fully capture the challenges of pruning LLMs. In this work, we address this challenge by introducing a straightforward and effective approach, termed Wanda (Pruning by Weights and activations). This technique successfully prunes LLMs to high degrees of sparsity without any need for modifying the remaining weights. Given a pretrained LLM, we compute our pruning metric from the initial to the final layers of the network. After pruning a preceding layer, the subsequent layer receives updated input activations, based on which its pruning metric will be computed. The sparse LLM after pruning is ready to use without further training or weight adjustment.
We evaluate Wanda on the LLaMA model family, a series of Transformer language models at various parameter levels, often referred to as LLaMA-7B/13B/30B/65B. Without any weight update, Wanda outperforms the established pruning approach of magnitude pruning by a large margin. Our method also performs on par with or in most cases better than the prior reconstruction-based method SparseGPT. Note that as the model gets larger in size, the accuracy drop compared to the original dense model keeps getting smaller. For task-wise performance, we observe that there are certain tasks where our approach Wanda gives consistently better results across all LLaMA models, i.e. HellaSwag, ARC-c and OpenbookQA.
We explore using parameter efficient fine-tuning (PEFT) techniques to recover performance of pruned LLM models. We use a popular PEFT method LoRA, which has been widely adopted for task specific fine-tuning of LLMs. However, here we are interested in recovering the performance loss of LLMs during pruning, thus we perform a more general “fine-tuning” where the pruned networks are trained with an autoregressive objective on C4 dataset. We enforce a limited computational budget (1 GPU and 5 hours). We find that we are able to restore performance of pruned LLaMA-7B (unstructured 50% sparsity) with a non-trivial amount, reducing zero-shot WikiText perplexity from 7.26 to 6.87. The additional parameters introduced by LoRA is only 0.06%, leaving the total sparsity level still at around 50% level.
NOTE: This text was largely copied from u/llamaShill
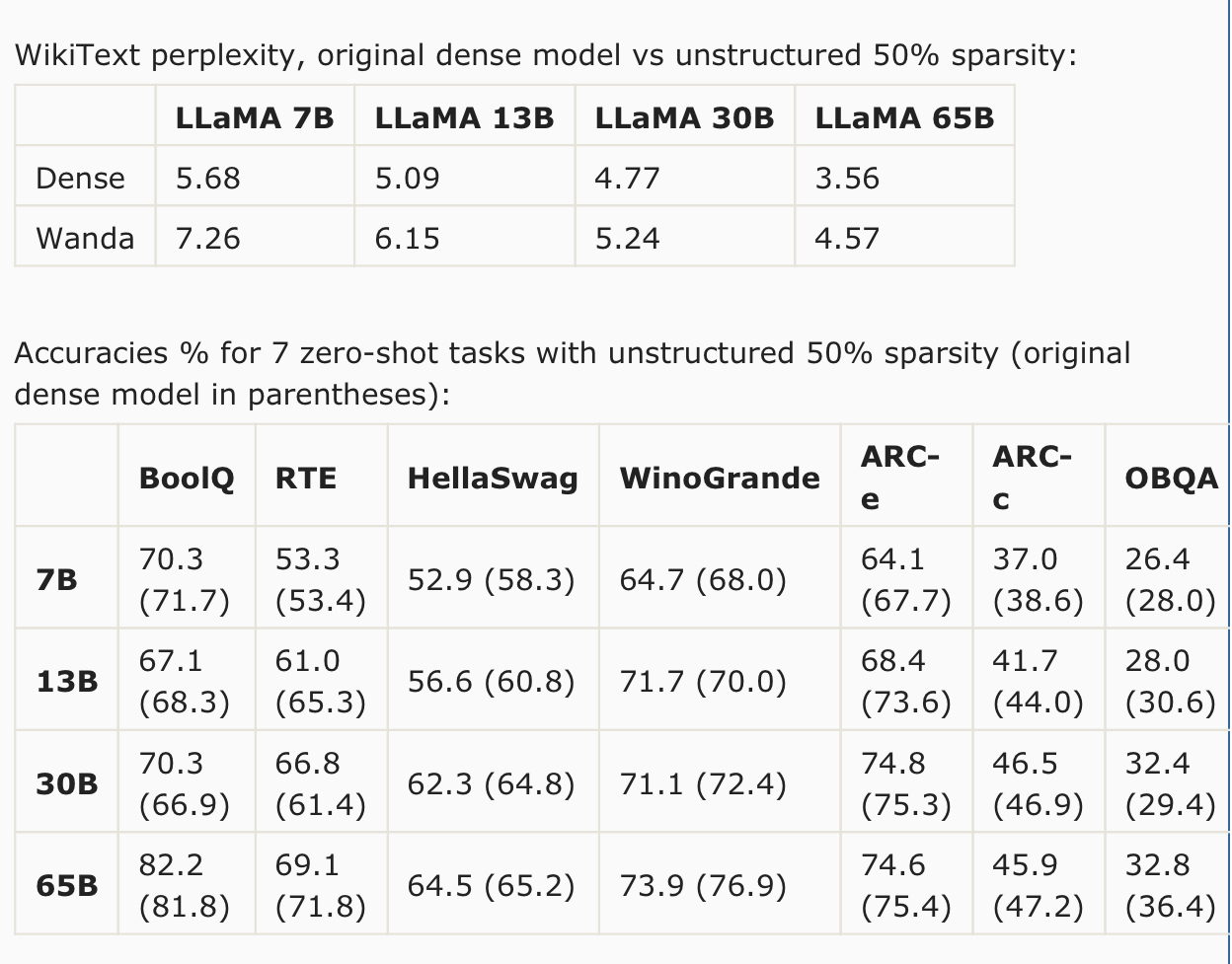
come out, maybe?